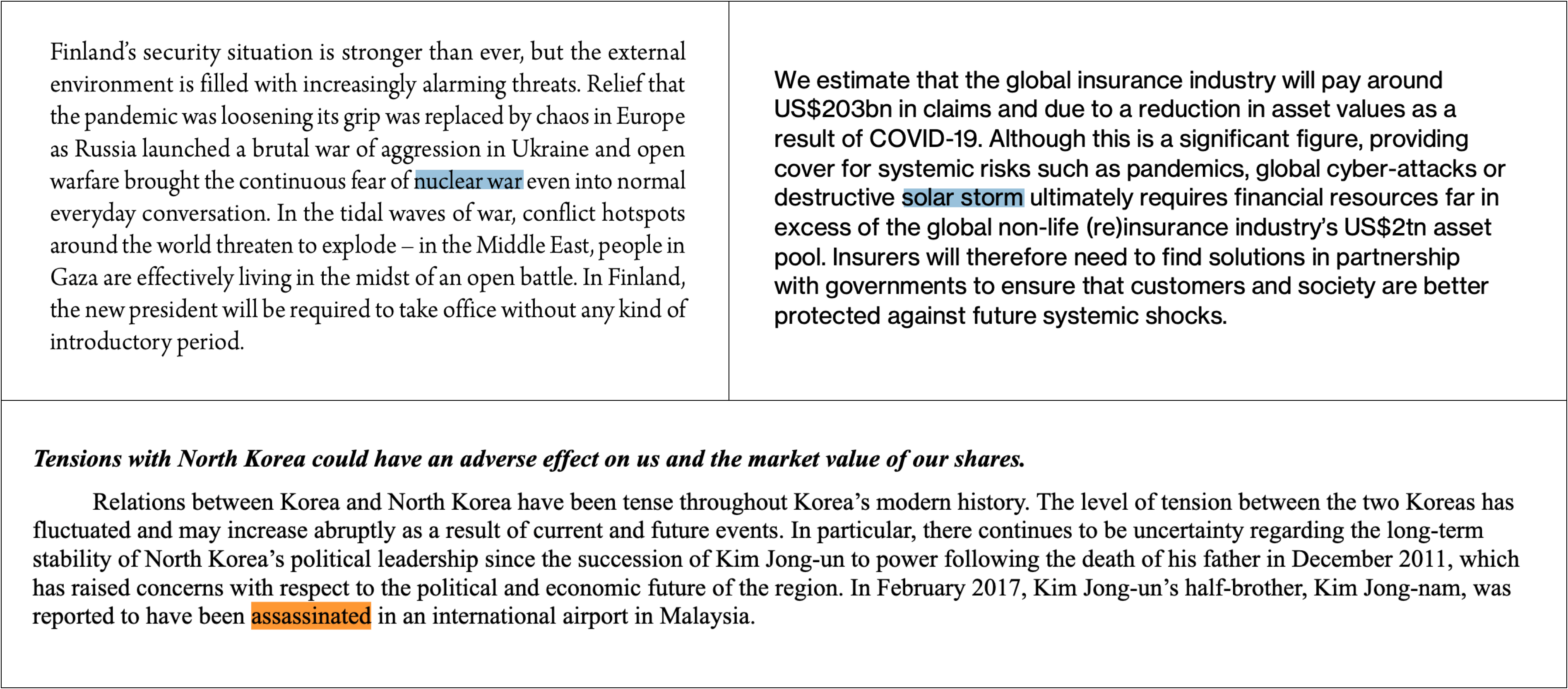
Corporate risk disclosures are often underappreciated as sources of valuable data with potential for creative application beyond finance and regulatory compliance. Wading through the jargon-heavy prose of annual reports can bring into focus industry-specific vulnerabilities and a spectrum of futures filtered through the attention of the corporation. Even the most extreme risks crop up within annual reports (Figure 1).
Crises continue to play an important role in strengthening the quality of risk data. The US public company disclosure system was founded in the aftermath of the Great Depression and the stock market crash of 1929. Fundamental transformations to risk disclosure were initiated following the collapse of Enron and the Dotcom bubble at the turn of the millennium. As a consequence of climate change and the increasing acceptance of climate risk as indistinguishable from financial risk, many companies are now required to publish detailed emissions data.
Whilst the quality of risk disclosure may be increasing, the format is heavy in detail, vast in scale and lacking in standardisation. The relentless volume of annual reports published each day challenges the attention of human analysts, investors and markets. Its therefore unsurprising to see the growing machine readership for annual reports. AI-driven software is being deployed to scour through risk disclosure for treasure.
There is treasure lying around waiting to be discovered. S&P analysts observed that investors and markets did not react to the inclusion of the following statement within Intel’s 2017 annual report: “if we face unexpected delays in the timing of our product introductions, our revenue and gross margin could be adversely affected”. This statement preceded a significant production delay to Intel’s 10-nanometer chips which caused a drop in share price. Stories of the future can be disguised as boilerplate. There are clear financial incentives to identifying such stories before humans and markets. There is also an abundance of increasingly sophisticated algorithmic approaches that can be tuned towards detecting these signals.
AI-driven software for analysing risk disclosure is predominantly developed within the financial services sector. If this were to remain the case, the applications of this technology are likely to remain focussed: detection of alpha signals, more accurate pricing or better prediction of market behaviour. However we believe that there is broader interest beyond the financial services sector in any project that could construct a detailed dataset of all the risks impacting the businesses that make up the UK’s economy.
AI-driven software could expand the scope of corporate risk monitoring. Analysts naturally focus on the UK’s largest companies but there is a long tail of companies beyond the FTSE whose risks remain underexplored.
Building upon these opportunities, our project is to develop risk monitoring software for exploratory research of the political economy. The software will feed into our research at Autonomy on changing working conditions by helping us to identify and analyse companies working at the frontlines of our unevenly distributed future. This might include companies situated in parts of the UK most vulnerable to extreme weather or those working within industries rebuilding disrupted supply chains.
Our first prototype for this project is called GERM (Geopolitical and Environmental Risk Monitor), a software tool for extracting geopolitical and environmental risks from reports filed electronically with Companies House. We used GERM to build a dataset of risks mentioned by the 266,989 UK companies who filed their accounts throughout March 2024. You can explore this dataset through an exploratory demo interface. In this post we will share the methodology guiding the development of this prototype. We also share findings on what risk data was discovered within Companies House throughout March 2024 and how we intend to develop this project further.
Methodology
Data
Companies within the UK file their annual reports at Companies House where they can be downloaded by the public (and machines). Extracting risk data from these documents with software is challenging for a number of reasons:
- Most companies don’t write about risks (sparsity)
- Any discussion of risk is usually scattered throughout the annual report (standardisation)
- Not all annual reports in Companies House are machine-readable (machine-readability)
Sparsity
The size of a company determines how much information it must include within any annual reports submitted to Companies House. Large companies will provide full accounts running into the hundreds of pages. There is likely to be some discussion of risk within these increasingly bloated documents that often exceed the length of the average novel. On the other hand small or very small companies are unlikely to produce reports that exceed several pages in length and will not contain any discussion of risk.
Standardisation
US companies disclose risks within the Item 1A - “Risk Factors"
section of their 10-K filings submitted to the Securities and Exchange Commission (SEC). The presence of a standardised section of text for risk discussion is obviously conducive to algorithmic analysis as any text in this section has essentially already been classified by the authors as relevant to risk. Unfortunately annual reports in the UK lack this kind of standardised risk reporting. Instead risk discussion can be found in multiple different sections of a report, such as strategy or the director’s remarks, although some reports contain sections like ‘Principal Risks and Uncertainties’. Any software trawling for risks is therefore required to search through the entire document rather than simply parse out a single section. Until recent advances in large language models (LLMs), this lack of standardisation made extracting risks particularly challenging.
Machine-readability
A fraction of companies do not submit annual reports to Companies House in a machine-readable format. Unfortunately this tends to be the largest companies like those that make up the FTSE. Instead these companies submit a scanned PDF file which is basically a collection of images. A machine-readable copy of these reports is often to be found via the FCA’s National Storage Mechanism or the company website. Medium to smaller companies are likely to file electronically such that their accounts can be downloaded as HTML files containing the machine-readable report with data labelled in the XBRL format. XBRL enables some of the data within reports to be easily extracted, like balance sheets and the number of employees.
Due to the lack of existing research on the risks reported by the UK’s medium to small companies coupled with the difficulties in curating machine-readable copies of the UK’s larger companies, our initial prototype is designed to monitor only the companies that file their accounts electronically. In 2022/2023 90.7% of companies filed their accounts electronically. 79.8% of the 3,857,049 companies that filed in 2022/2023 are considered to be small or very small companies as they filed their accounts under the categories of micro entity, audit exempt or small. We therefore require GERM to process a large number of companies (potentially tens of thousands of reports per day) that are unlikely to contain any discussion of geopolitical or environmental risk.
Risk Quantification
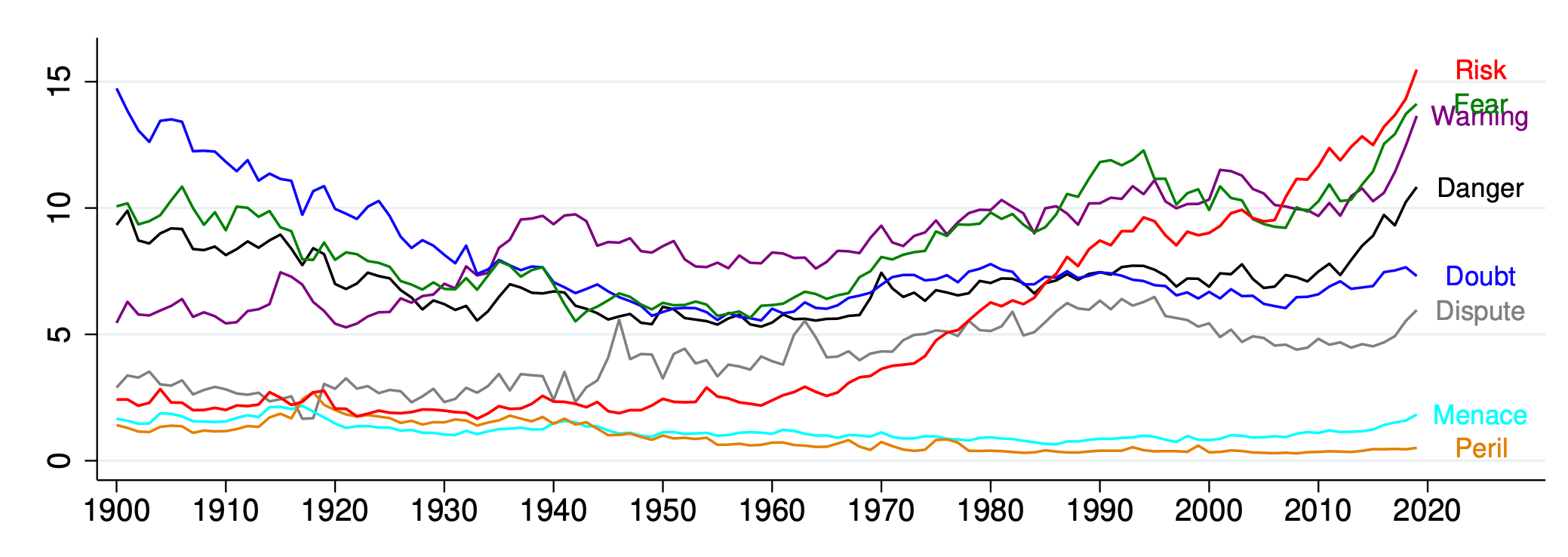
Whilst developing GERM, there were several examples of software for visualising risk across large corpuses of unstructured text that informed our thinking.
US Federal Reserve economists Dario Caldara and Matteo Iacoviello developed a text-based geopolitical risk (GPR) index that mines news articles for combinations of well-chosen keywords correlating to geopolitical risk. The sources of news that feed the index, including the Financial Times, The New York Times (Figure 2) and The Wall Street Journal, have extensive digital archives allowing the researchers to test how well their index captures historic events. The index tends to spike in times of war and peaked on 9/11.
Blackrock developed a comparable geopolitical risk indicator for measuring ‘market attention’ towards 10 of the top geopolitical risks as they see it. As of April 2024 they are closely tracking the potential for a Russia-NATO conflict, gulf tensions and major terror attack(s). The index combines the outputs of machine learning models fine-tuned to detect relevance to each risk topic and sentiment.
Compared to text-mining, the use of more advanced language models allows for a greater level of context to be factored into any classification of unstructured text. This expanded capability invites the need for some constraints to guide the development of novel risk monitors. After all what risks are we interested in tracking? When designing prompts to guide an LLM, how can we keep the language sufficiently adaptive so as to account for emerging risks? LLMs may be able to highlight sections of text discussing risk but in aggregate we still require some degree of classification to understand if war is being reported on more than supply chain disruption or drought. This suggests the need for a good taxonomy.
Taxonomy
Taxonomies form the corner stone of corporate risk strategy. They establish a common language for describing risk. Consultants might say “if it is in the risk taxonomy, it gets managed.” Some criticisms levelled at these frameworks include the failure to sufficiently incorporate emerging risks or black swans. For our project we are looking to identify geopolitical and environmental risks but don’t actually know what subsets of these broad genres of risk might be found in Companies House. How many references to the ongoing war in Palestine might we encounter daily? Would any companies be impacted by earthquakes abroad? We are really looking to use a taxonomy as a tool for discovery. Something to draw upon when contructing a series of flexible prompts to guide an LLM in filtering data. Therefore we sought out the most extensive taxonomy possible. This led us straight to the Cambridge Taxonomy of Business Risks (Figure 3).
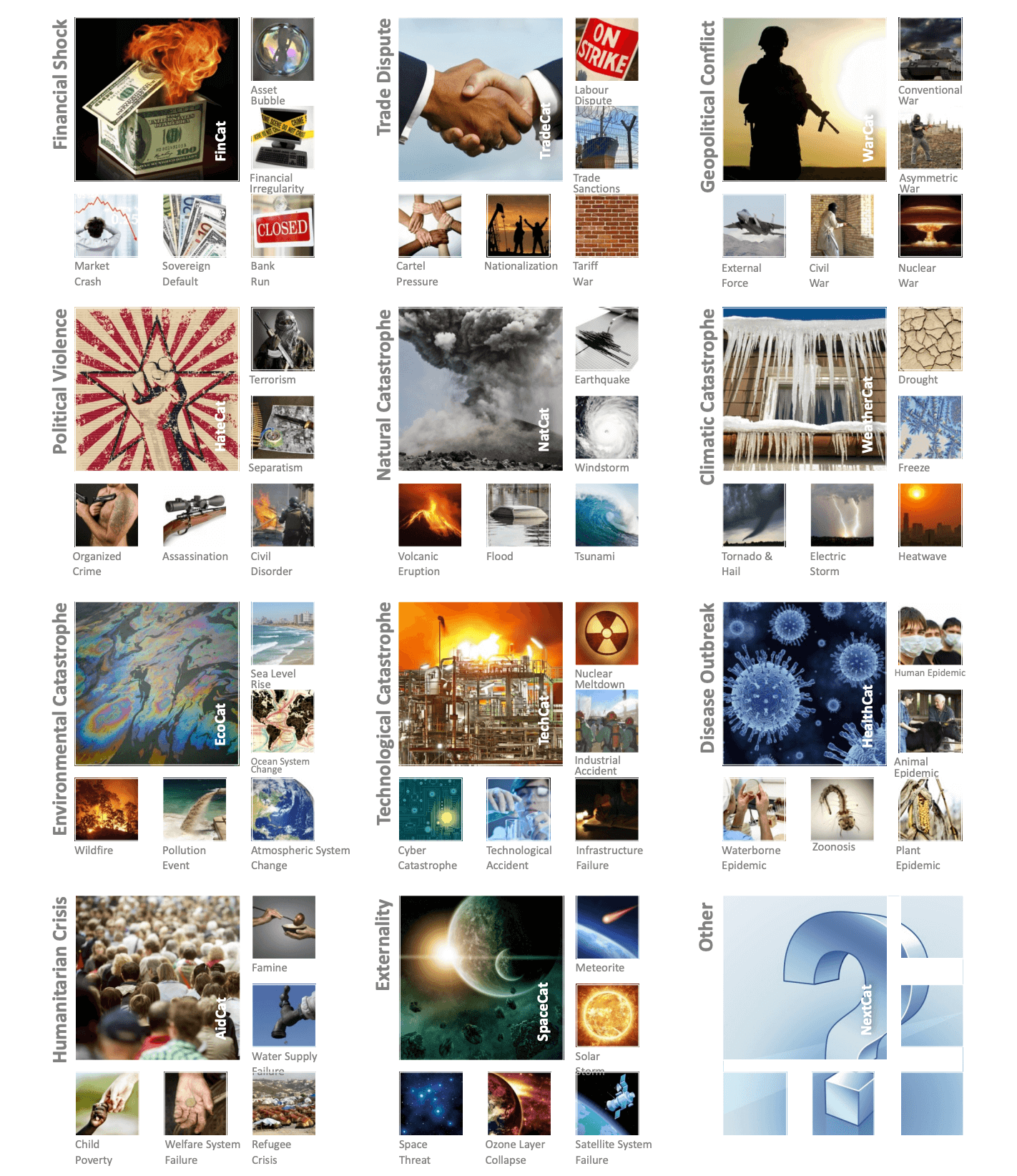
Prototype
Building upon the preceding research, we developed the first prototype for GERM to visualise the geopolitical and environmental risks flagged within annual reports filed electronically with Companies House across the month of March 2024 (Figure 4).
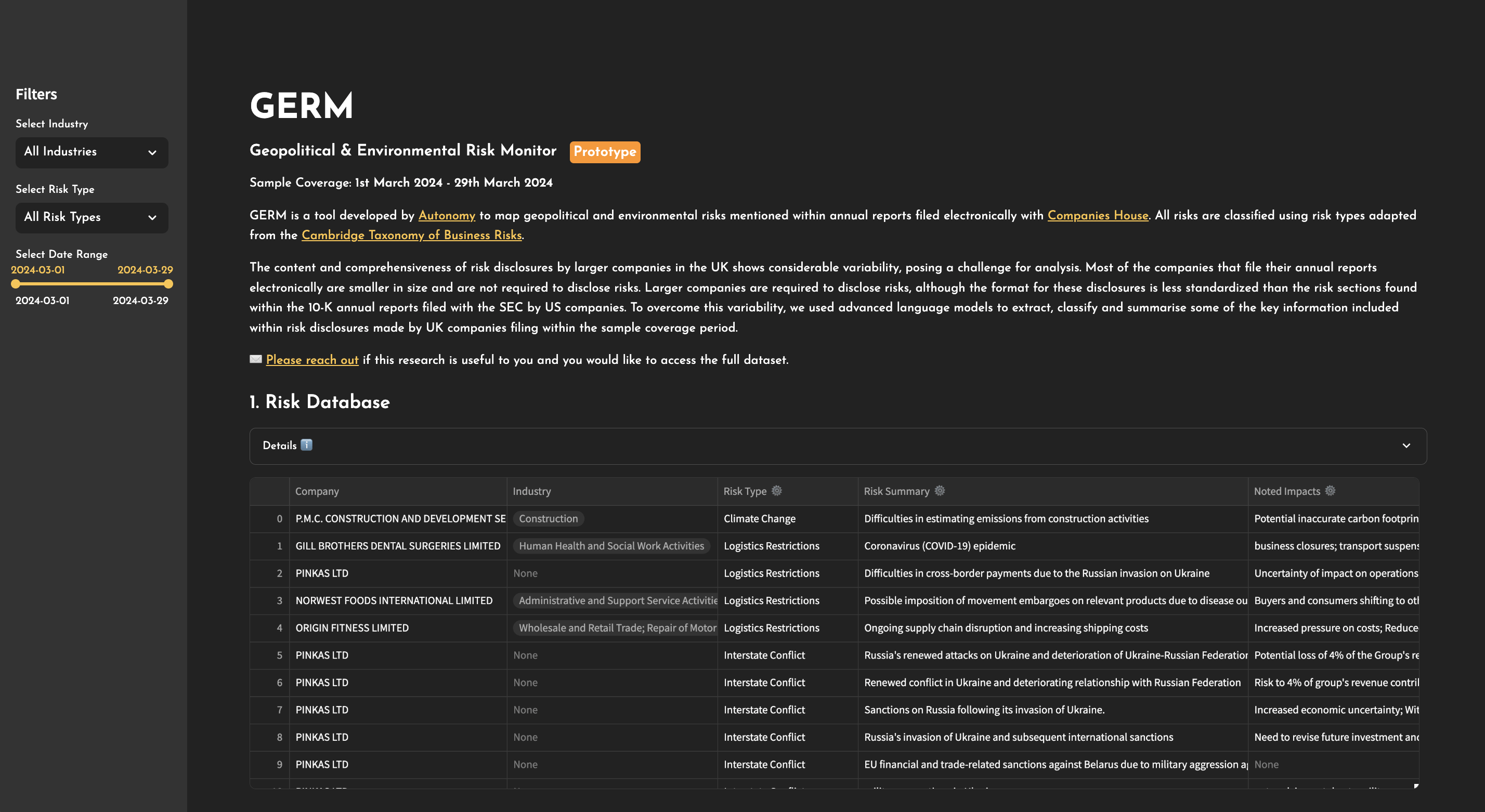
GERM classifies each risk using a selection of categories adapted from the Cambridge Taxonomy of Business Risks. Our adapted taxonomy takes the following shape:
Geophysical
Risk Type | Description |
---|---|
Change in Government | A shift in political and social ideology or change in leadership that has disruptive impacts on existing business practices |
Corruption Deterioration | The abuse of power for personal gain through bribery, nepotism, kleptocracy etc. |
Criminal Activity | Significant crimes committed against the business or its customers |
Emerging Regulation | Upcoming regulation or policy changes |
Industrial Action | Widespread discontent embodied by strikes, riots, civil commotion and protest or slowdown |
Interstate Conflict | Armed or unarmed combat among nation states |
Logistics Restrictions | Bottlenecks or limits of access to key global transport routes |
Minimum Wage Hike | Prevalent increase in minimum wage rates across demographic groups |
Modern Slavery | Individuals forced to work, trapped and controlled by an “employer” through abuse, dehumanisation and containment |
Nationalisation | Transformation of private assets under public ownership with or without compensating the former owners |
Privatisation | Sale of state-owned businesses to private investors, or private entities become responsible for implementation of government programmes or services |
Sanctions | International sanctions regimes, geo-economics, trading blocs, bi/multi-lateral negotiations and disputes, court penalties, trade bans or other coercive measures within or between nation states out of political reasons |
Social Unrest | Mass acts of civil disobedience (e.g. demonstrations, riots) where the participants become hostile towards the authorities, and the authorities have difficulties in maintaining public safety and order |
Subnational Conflict | Localised regional separatism through to large-scale, armed violence between organised groups within the same state or country, typically to change leadership, damage public safety and order |
Talent Availability | Skills shortages |
Terrorism | Unlawful use of violence and intimidation against civilians for political reasons, perpetrated by individuals or groups inspired by domestic or international extremist ideologies |
Environmental
Risk Type | Description |
---|---|
Climate Change | Acute and/or chronic physical hazards associated with long-term changes to the Earth’s climate, as well as risks posed by society’s responsive transition to a low carbon economy |
Environmental Degradation | Deterioration of the physical environment and ecosystems, including: waste & pollution, biodiversity loss, ecosystem collapse, deforestation & soil degradation |
Extreme Weather | Weather events that impact business: flooding, tropical storms, heavy precipitation (rain, hail, snow), lightning storms, drought, freezing and low temperatures, heatwaves, wildfires etc. |
Food Security | Shortages of food affecting large populations due to environmental factors and/or disease outbreaks in plant and livestock food sources |
Geophysical | Earthquakes, tsunamis and volcanic eruptions |
Natural Resource Deficiency | Deficiencies in natural resources caused by unsustainable human consumption at a rate exceeding the readily available supply, including: fossil fuels, biogeochemicals, raw materials, water etc. |
Space | Solar storms and astronomical impact events |
GERM’s Risk Monitoring Pipeline
GERM processes annual reports through a series of steps. Documents are downloaded and the text within them is extracted and separated into chunks. Each chunk of text is searched for keywords associated with each category of risk within the taxonomy (Figure 5).
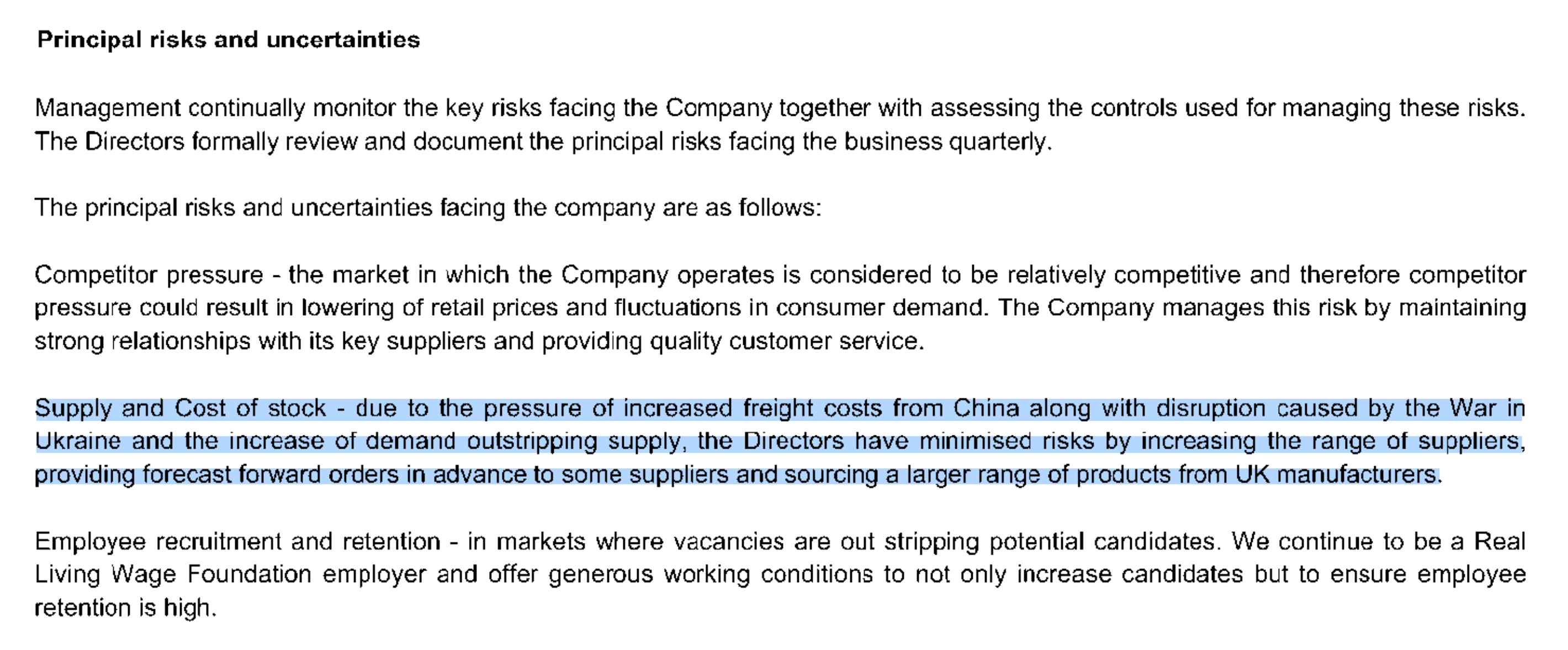
Any chunks containing keywords relevant to a category of risk within our taxonomy proceeds to be processed by an LLM that classifies the chunk as to whether or not it contains discussion relevant to the flagged category of risk.
If the LLM flags the chunk as containing relevant discussion pertaining to the category of risk, the chunk proceeds to be processed by a sequence of LLMs that summarise the risk discussion, extract and summarise stated impacts and extract the names of any countries mentioned. This is the data that ultimately populates the risk database where it can be searched for keywords, sorted and downloaded (Figure 6). This pipeline is biased towards higher precision at the expense of recall so there are likely to be instances of risk reporting that are not flagged as such.

Risk Impact Embeddings
Each risk impact, such as loss of staff
, increase in costs
, or damage to building
, is processed by an embedding model that generates a semantic embedding vector for each description. Within GERM these vectors are represented in a semantic space—a kind of map where each point (vector) represents the meaning of a risk impact (Figure 7). The closer two vectors are on this map, the more similar they are in meaning. For instance, in two-dimensions, the vector for loss of staff
might be positioned closer to the company struggles to retain employees
than to crops were damaged by drought
. This mapping allows us to visually identify and analyse:
- Different types of risks leading to similar impacts on different companies. This is shown as clusters of mixed colour points on the map. For example,
extreme weather
,interstate conflict
, andfood security
might all cause similar increases in farming costs and prices. - The most frequent impacts associated with each type of risk on different companies. This is shown as clusters of points of the same colour. For example,
interstate conflict
often results insupply chain disruptions
,rising energy costs
, andinflation
.
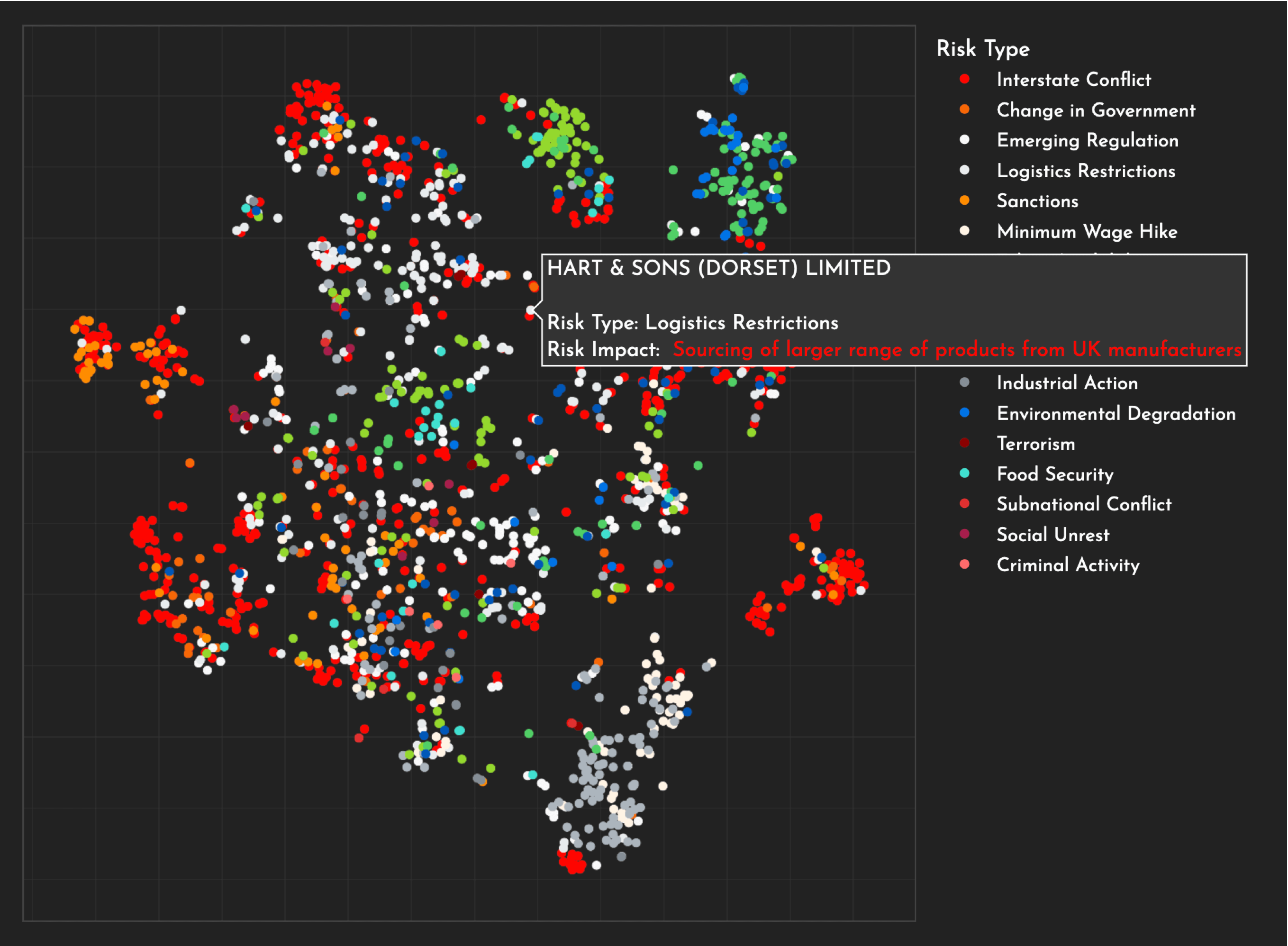
The extraction of named entities in the form of countries can serve as input to a global risk heatmap visualising the countries most frequently appearing within risk disclosures (Figure 8).
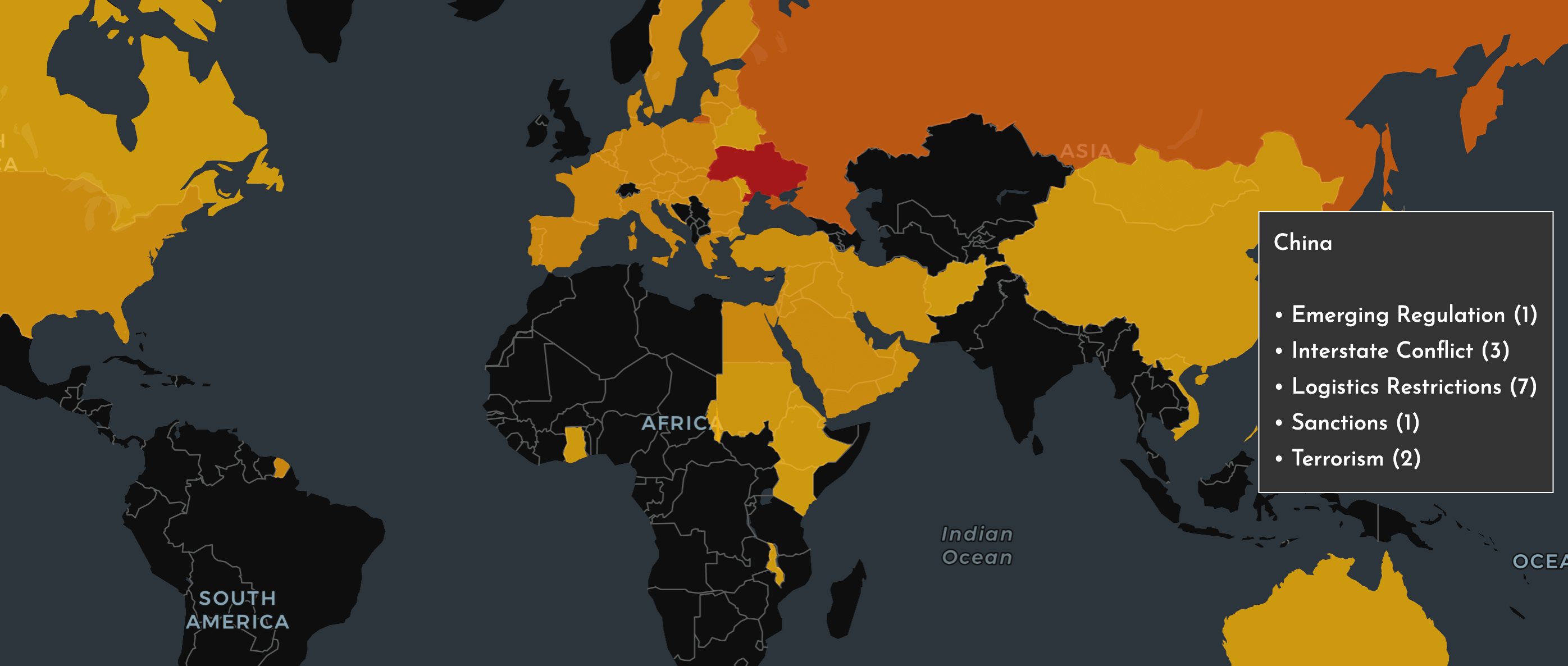
March 2024 Observations
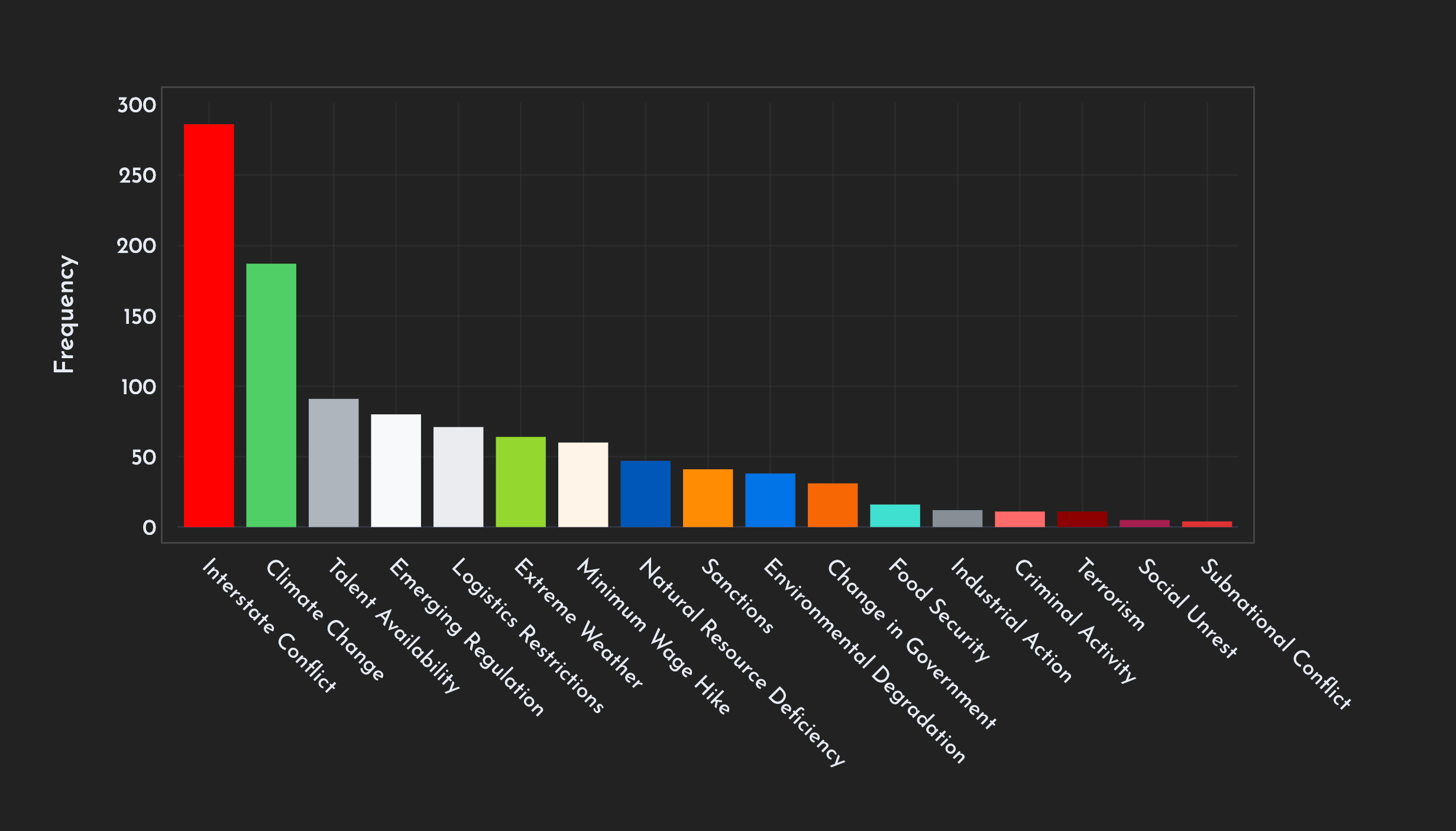
Of the 266,989 annual reports processed by GERM throughout March 2024, only 621 companies were flagged as reporting any of the risks from our taxonomy. As suggested within our methodology, the majority of companies filing electronically will be smaller in size and are therefore less likely to include detailed risk disclosure. Some of the largest companies reporting relevant risks within the month included Rapiscan Systems (airport security hardware specialists), FP Mccann Group (supplier and manufacturer of precast concrete) and Enerveo (contractors).
Discussion of interstate conflict and climate change appeared more frequently that other types of risk, with the wars in Ukraine and Palestine continuing to focus attention (Figure 9). Climate change risks were often flagged by GERM when companies share emissions data under the Streamlined Energy and Carbon Reporting guidelines. Some categories of risk were not detected in any reports throughout the month including privatisation, nationalisation and space risks. Some risk types including modern slavery and corruption deterioration returned similar boilerplate compliance statements and were therefore omitted as they provided minimal information.
Trends in risk reporting across each category of risk could be summarised as follows:
Risk Type | Risk Trends | Impact Trends |
---|---|---|
Change in Government | UK & US upcoming elections in 2024 | Economic uncertainty; Changes in government funding for specific sectors; Reduced consumer confidence |
Climate Change | GHG emissions (Scope 1 & 2); High energy consumption; SECR compliance | Financial commitments to Net Zero; Increased costs of optimising processes, sustainable materials and carbon reducing technologies; Challenges in meeting the changing preferences of carbon conscious consumers |
Criminal Activity | Young people getting involved in crime and anti-social behaviour; Fraud | Implementation of new policy; Repayment of illegal dividends |
Emerging Regulations | Brexit | Increased costs and financial pressure; Increasing trade friction and complexity; Reduced business with the EU; Lobbying efforts advocating for legislative change |
Environmental Degradation | Challenges in sourcing of sustainable materials; Use of landfills for waste management; Risk of dangerous leakage into the environment | Increased costs due to recycling and sustainable materials; Reputational risk |
Extreme Weather | Flooding; Heavy rain; Drought; Extreme heat | Crop damage; Delayed planting; Increased importation; Investment in new technologies; Damage to infrastructure |
Food Security | Avian influenza | Operations impacted; Loss of food and lifestock; Rising operational costs |
Industrial Action | Entertainment industry strikes; UK rail strikes | Challenging business conditions; Challenges in travelling by rail; Uncertainty regarding planned events |
Interstate Conflict | Wars in Ukraine and the Middle East | Inflation; Energy price rises; Supply chain disruptions; Market volatility; Raw material costs increased |
Logistics Restrictions | Global conflicts; COVID-19 government restrictions; Suez Canal; Houthi strikes in the Red Sea | Increased shipping and transportation costs; Extended lead times; Redesign of supply chains |
Minimum Wage Hike | 10% Increase to the National Minimum Wage; Commitment to Real Living Wage; Wage inflation | Significant rise in payroll and labour costs; Decrease in profit; Pressure on employee retention and recruitment efforts |
Natural Resource Deficiency | Volatile raw material prices (fossil fuels and metals); Energy crisis; Brexit and global conflict impacting raw material availability and prices; Increased costs of sustainable materials | Increased or unpredictable production costs; Investment in renewables (solar) and recycling |
Sanctions | EU, US and UK sanctions against Russia and Belarus; Potential future sanctions against China; Ban on Western airlines flying within Russian airspace | Challenges in accessing funds and customers; Revenue loss from the Russian market; Potential difficulties for companies to continue operating; Future uncertainty around inclusion on sanctions list |
Social Unrest | London protests; Instability in Ethiopia and Syria | Projects and travel abroad delayed or suspended |
Subnational Conflict | Civil war in Sudan | Negative impact on Exotic Debt Fund; Negative impact on existing projects and company performance |
Talent Availability | Brexit-induced labour challenges; Skill shortages in construction, nursing and technology | Wage inflation and increased labour costs; Opening of Tier 2 visa sponsorship schemes to attract talent from the EU and elsewhere; Investment in recruitment and in-house training; Continued support for apprenticeship schemes |
Terrorism | Increasing alertness towards terrorist threats in public spaces such as sports stadiums | Financial, care and social support required for victims of terrorism; Potential for a reduction in international travel following terrorist attacks |
A closer reading of GERM’s dataset reveals some eye-catching risks worth digging into further:
Geopolitical
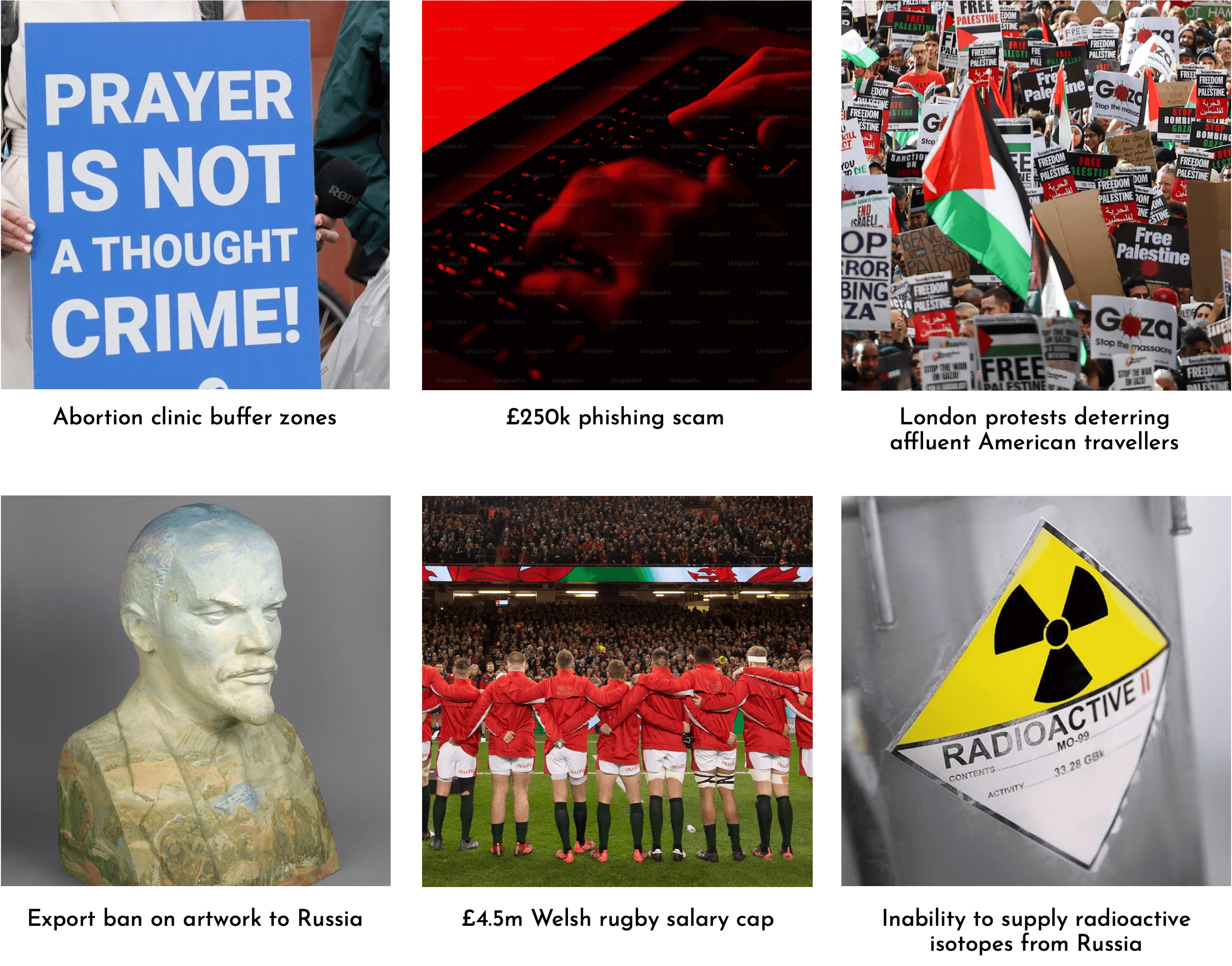
Environmental
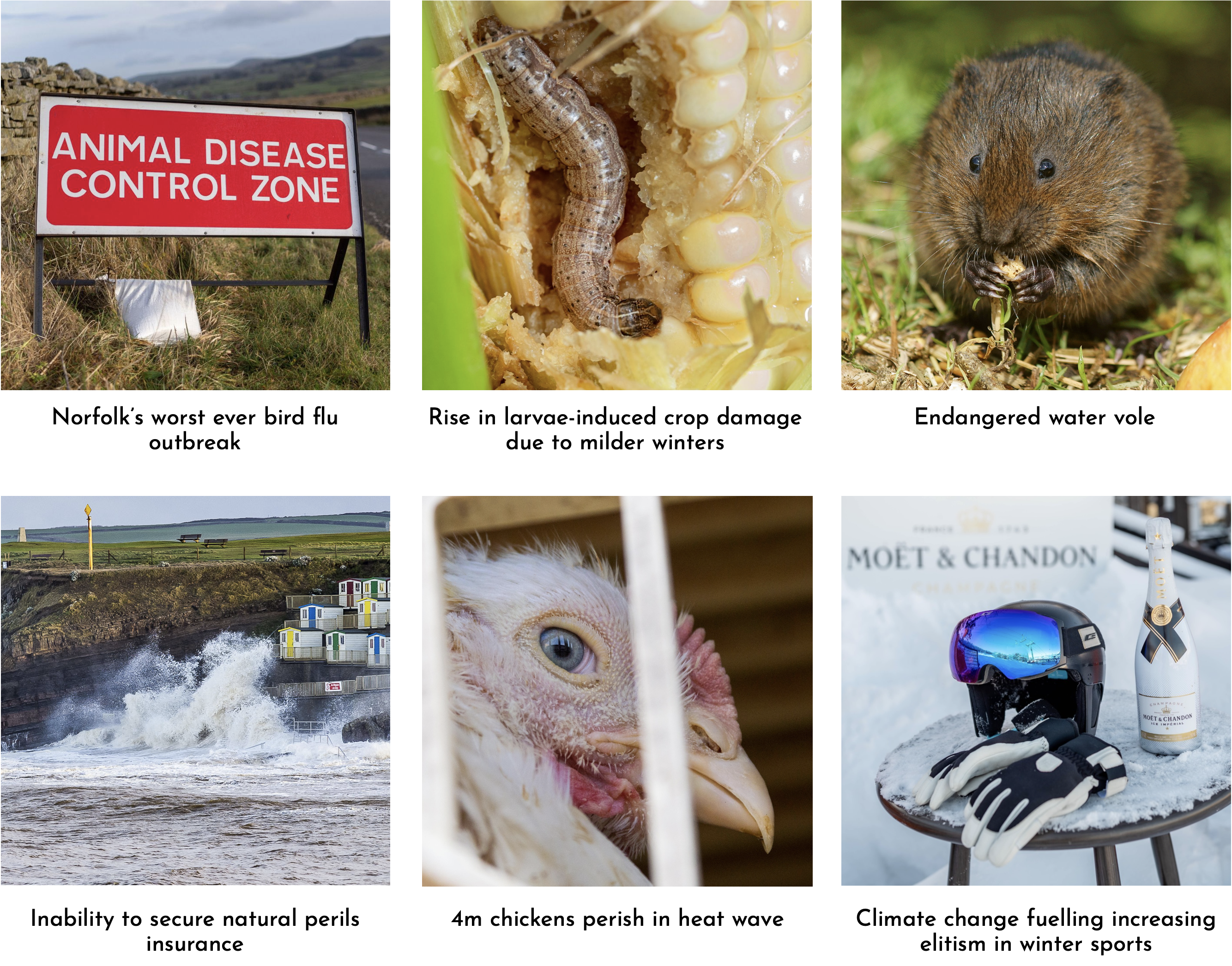
GERM also flagged instances of risk that could work out to be advantageous for certain companies. Kabina own the patent for an amphibious flood-adaptive home and write in their annual report that “the UK’s relentless and exponential increase in population, combined with increased areas of flood land, bode well” for their business (Figure 12). Mercian Limited, the UK’s largest supplier of crisping potatoes, recorded their best financial year yet as a consequence of drought and the war in Ukraine leading to a hike in the price of potatoes.
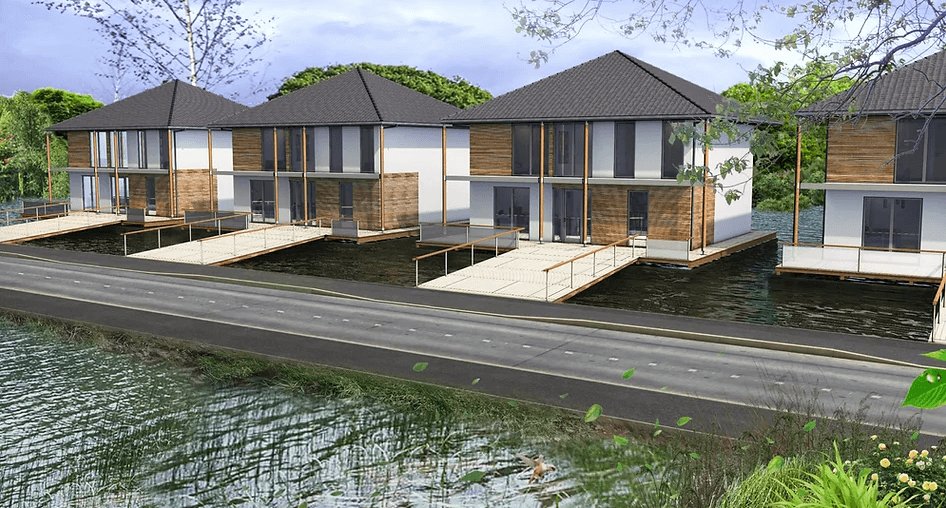
AI-Augmented Risk Research
As we continue to experiment with GERM within our broader practice of prototyping AI-augmented research tools, there are several language model specific research directions on the horizon that we look forward to developing.
Islands of Coalition
By generating and mapping embedding vectors of risk impacts, we can visualize clusters of companies experiencing similar challenges (Figure 13). This map may reveal unexpected clusters of companies, separated by locality and industry, that could unite around similar risk-mitigation policies. We look towards other coalitions such as the Alliance of Small Island States that illustrate how entities from different cultures and contexts can find common purpose in response to shared risk. How could uncovering further islands of latent coalition inform policy design?
Advanced Concept Filtration
Given the advanced comprehension of LLMs and the ease with which text processing operations can be chained together to compose complex pipelines, we are curious to explore how well LLMs could filter Companies House for more sophisticated concepts of risk and resilience beyond those within the Cambridge Taxonomy of Business Risks. Could we identify instances of companies that exhibit ‘antifragility’ by thriving in challenging business environments. Other more nuanced phenomena to monitor for might include ‘climate change adaptation measures’, ‘supply chain diversification’, and ‘business model innovation’.
Companies House for Interactive Planning Simulations
Most companies don’t write about risk. Most risk management professionals will have considered using ChatGPT to fill in the blanks of a risk report. What remains less explored is how far we could push the obvious potential for LLMs to generate risk profiles for companies. Annual reports could be processed to infer the level of exposure each company has to every single category of risk within the Cambridge Taxonomy (even the most Emmerichian risks). Coupled with external data from news, reports, social media and fiction, LLMs could generate plausible narrative-based scenarios detailing how companies might respond to each flavour of crisis. These narratives could form their own labyrinthian genre of grey-literature; a house of generative risk narratives for each company in Companies House. Elements of this data might serve as input to an agent-based-model for simulating and studying the emergent competition and cooperation within fragments of the economy under stress. Could Companies House serve as the partial backend database to simulations for incubating and archiving novel plans and strategies?
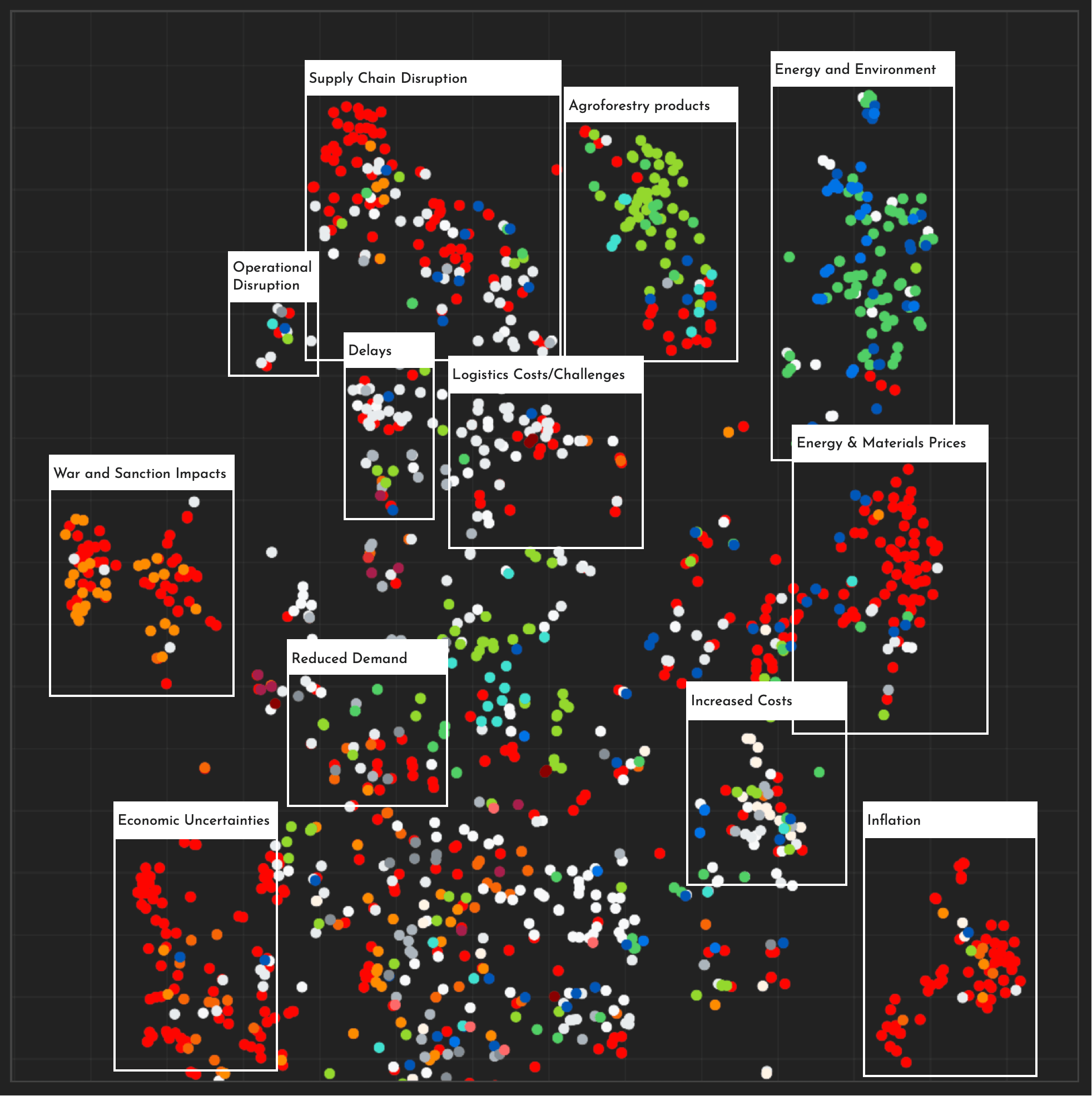